Total of Funding Awarded $7.7M per year – Twelve Selected Projects
Fostering Innovations for Community Modeling
WPO launched the Innovations for Community Modeling Competition in FY23 to provide support groundbreaking projects that enhance scientific understanding and forecasting through innovation. The competition selected 12 projects from academia, government, and industry with a focus on advancing the Unified Forecast System (UFS) to become the world’s most accurate and reliable operational modeling system.
The Innovations for Community Modeling initiative integrates priorities from four WPO programs – Air Quality & FIre Weather (AQFW), Earth Prediction Innovation Center (EPIC), Joint Technology Transfer Initiative (JTTI), and Subseasonal to Seasonal (S2S) – funding projects within three program areas:
- Numerical Weather Prediction (NWP) Capabilities
- Western States Hydrology
- Fire Weather Subseasonal-to-Seasonal (FW-S2S) Forecasting
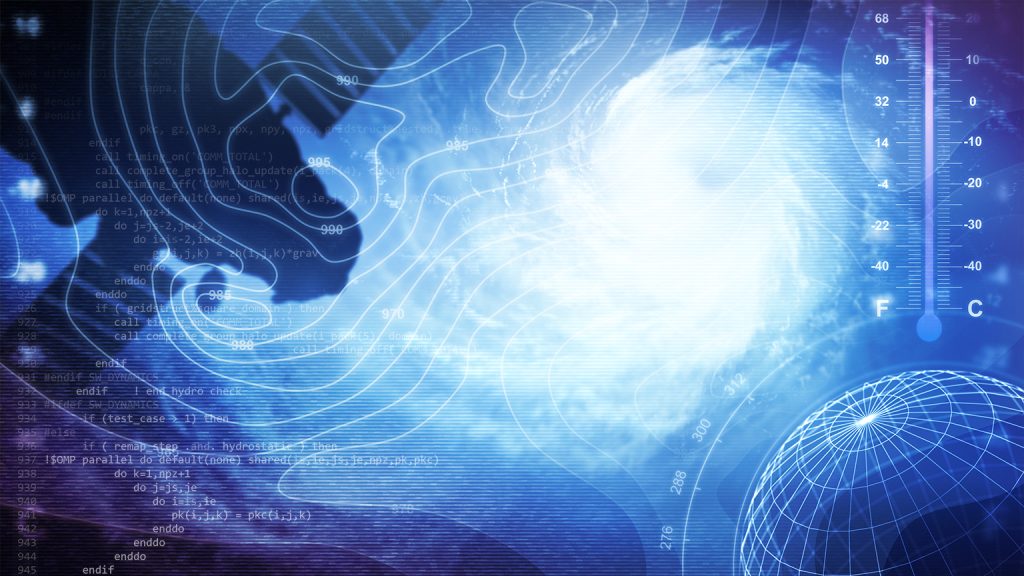
Program Area I: Numerical Weather Prediction (NWP) Capabilities
Projects under the Numerical Weather Prediction Capabilities program area support the development and advancements of the UFS to become a fully-coupled Earth prediction system, addressing all scales from the mesoscale short range to the seasonal-to-subseasonal predictions across the linked weather-water-climate domain. Envisaging high-risk/high-reward opportunities that may accelerate the development of such capabilities in the UFS, projects funded within this program area will develop next-plus-1-generation capabilities in component models or systems, such as atmospheric physics or dynamics, coupled data assimilation, ocean, ice and/or land systems, for example.
The seven projects in this area will receive two-year awards via cooperative agreements with $3,763,057 total funding, consisting of $1,916,870 from the JTTI Program and $1,846,187 from WPO’s Director’s Fund.
*Award totals are distributed over the life of the projects and conditional on appropriations
Projects Selected
Project Title | PI’s /Co-PI’s | Project Description |
---|---|---|
Non-Gaussian Ensemble Data Assimilation within a Rapidly Updating Global Prediction System | Jonathan Poterjoy (University of Maryland, CIRES/CU Boulder) PI Laura Slivinski (University of Maryland, CIRES/CU Boulder) PI | The project will evaluate non-Gaussian data assimilation in a rapidly updated global modeling system, investigate the impact of Gaussian assumptions on forecasting uncertainty, integrate new data assimilation infrastructure into JEDI, and include a global assessment of precipitation bias within a framework that is easier than with current operational systems. |
Improving warm-rain moist physics in the Unified Forecast System | Clifford Mass (University of Washington) PI Robert J.C. (University of Washington) PI | This project aims to improve warm rain forecasts in the NOAA Unified Forecast System (UFS) through the RCON approach. The aim is to enhance hydrometeorological forecasting and save lives while safeguarding property in diverse geographical regions of the western United States. |
Impact of Stochastic Parameterizations on UFS Biases and Seasonal Forecast Errors | Prashant. D. Sardeshmukh (CU Boulder) PI Gilbert P. Compo (CU Boulder) PI J.-W. Aaron Wang (CU Boulder) PI Cecile Penland (CU Boulder) PI | This project aims to investigate the impact of stochastically-generated changes on a model’s biases and the improvement of seasonal forecasts. The investigation will involve analyzing existing large-ensemble simulations, specifically looking at the mean climate, climate variability, and extreme weather statistics in the FV3GFS model. |
A machine learning postprocessor to mitigate QPF errors for improved hydrometeorological forecasting | Kristie J. Franz (Iowa State University) PI William A. Gallus, Jr. (Iowa State University) Co-PI | This project aims to improve NOAA forecasting by utilizing machine learning (ML) to develop a post-processing technique for Quantitative Precipitation Forecast (QPF). |
Developing Novel, Robust Hybrid Machine-Learning Postprocessing Schemes for Improving Skills of UFS Probabilistic Forecasts of Precipitation Amounts and Types | Yu Zhang (University of Texas Arlington) PI | This project aims to develop innovative machine learning (ML) algorithms for ensemble post-processing, specifically focusing on generating skillful probabilistic guidance for precipitation amounts and types. |
Unification of Boundary-Layer and Shallow Cumulus Mass Fluxes under Vertical Wind Shear for the Unified Forecast System Models | Xiaomin Chen (University of Alabama Huntsville) PI George H. Bryan (NCAR) PI Andrew T. Hazelton (University of Miami) PI | This project aims to develop a generalized Eddy-Diffusivity Mass Flux (EDMF) scheme for the Planetary Boundary Layer (PBL) that unifies the mass flux parameterizations for clear and shallow cumulus boundary layers. The project involves code development, LES and SCM tests, parameter analysis, and implementation into the Hurricane Analysis and Forecast System (HAFS) for retrospective runs during the 2024 hurricane season. |
Developing enhanced diagnostic output for the UFS: Increasing information extraction with minimal expense | James Correia Jr (CU Boulder) PI Larissa Reames (University of Oklahoma) PI | The deliverable is expected to support improved forecasts by enhancing the understanding of model behavior during development, enabling forecasters to focus on real model extremes instead of numerical artifacts, and facilitating better monitoring of extreme model behavior in operational deployments. |
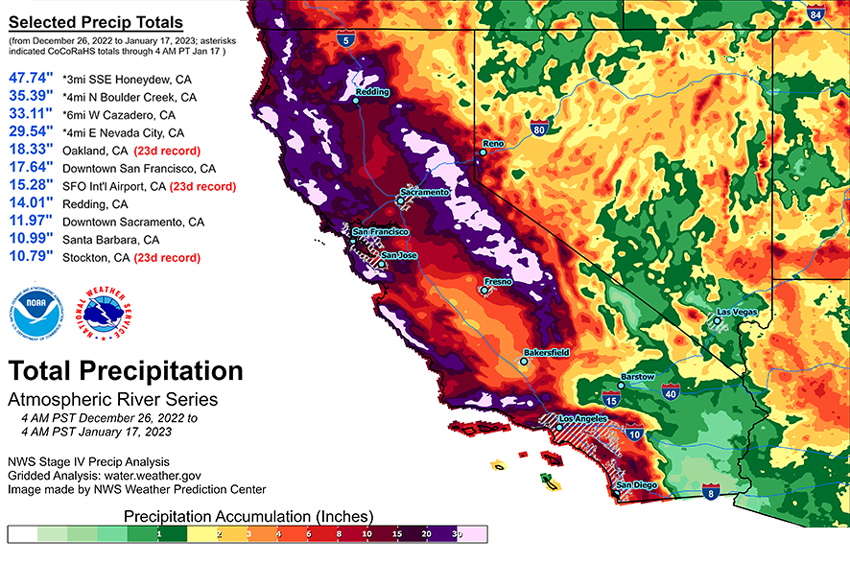
Program Area II: Western States Hydrology
NOAA is striving to enhance its ability to predict weather patterns over periods ranging from a few weeks to two years to fulfill its commitment to the Weather Research and Forecasting Innovation Act of 2017. Funded projects in this program area aim to improve precipitation and hydrology forecasts, particularly for the Western states. The objective is to use a unified approach to modeling that incorporates different factors that influence weather patterns and extremes, including cyclical modes of variability and their impact on hydrology in the region. Selected projects bring several innovations to improve predictions related to the hydrologic cycle, such as precipitation patterns, streamflow, and interactions between the land and the atmosphere, with the goal to enhance the accuracy of hydrological predictions in the Western states via improved numerical modeling processes and data assimilation.
The three projects in this area will receive two-year awards via cooperative agreements with $2,335,066 total funding from the S2S Program.
*Award totals are distributed over the life of the projects and conditional on appropriations
Projects Selected
Project Title | PI’s /Co-PI’s | Project Description |
---|---|---|
Use of Analysis Increments to Advance S2S Predictions of Western States Precipitation Through Targeted Process-Level Improvement of the MJO in the Next-Generation NOAA GEFS | Stefan Tulich (CU Boulder) PI Jian-Wen Bao (NOAA PSL) PI | The challenge of reliably predicting precipitation patterns and their extremes across the western US at lead times beyond two weeks is of obvious interest to stakeholders in the region. A major obstacle in this regard, however, is the inability of current operational models to faithfully capture known sources of potential prediction skill. These sources include the 30-60-day Madden-Julian Oscillation (MJO), whose poor simulation in the NOAA Global Forecast System version 15 (GFSv15) has been linked to errors in that model’s forecasts of precipitation along the US West Coast at Weeks 3–4. Here we propose to address this issue in the context of the next-generation NOAA Global Ensemble Forecast System version 13 (GEFSv13), which is currently under development and will be a fully coupled atmosphere-ocean model, unlike its predecessor. The proposal fits well within the scope of the Western States Hydrology Program of the FY23 NOAA/OAR/WPO Innovations for Community Modeling Competition. In particular, by using analysis increments to advance S2S predictions of western states precipitation through the targeted process-level improvement of the MJO in the prototype coupled GEFS, the project will “identify and address the sources of model biases” in order to “better harness predictability sources” that are “most relevant in the Western States Hydrology program”. |
Advancing UFS forecast model evaluation and improvement for S2S hydrometeorological prediction in the Western States | Andrew Newman (NCAR) PI Yifan Cheng (Univ. of Arizona) PI | This project aims to demonstrate improvements in UFS subseasonal precipitation and year-round hydrology forecast skill across the Western US. The focus is on enhancing process-level understanding within the Noah-MP land model and land-atmosphere coupling, providing insights into the causality within the coupled model. The primary target audience for the forecast innovations is the NOAA/NCEP/EMC land group, with the goal of advancing the greater land-modeling community. Improved UFS forecast skill in weeks 2-5 benefits the Climate Prediction Center (CPC) and all users of NOAA forecasts, ultimately benefiting the nation’s economy and livelihoods. The deliverables include peer-reviewed publications, documentation, presentations, and public releases of data and analysis code, providing valuable information on process-level coupling biases and optimization methodologies within Noah-MP. |
Exploring concerted integration of alternative snow parameterization and novel interactive vegetation phenology to improve UFS S2S predictions of snowpack and drought termination over the western US | Yu Zhang (Univ. of Texas Arlington) PI Lixin Lu (Colorado State Univ.) PI | Over the past decade alone, the western and southwestern US experienced intense droughts and devastating floods that have profound economic consequences for the nation. Accurate predictions of these events at subseasonal-to-seasonal (S2S) scale have far reaching implications for water management over these regions, and yet have been challenging to attain. Of the many contributors to the lack of S2S forecast skills, inadequacy in the representation of surface conditions by land surface models (LSMs), as well as its downstream impacts, have yet to gain adequate attention. Past research by the project team on Noah-MP, the designated LSM for UFS, has underscored several critical deficiencies, including artificially fast snow sublimation and melt, and timing errors in the seasonal cycle in vegetation phenology and soil water uptake. The former leads to persistent underestimation of snowpack, and the latter distorts root-zone soil moisture dynamics, evapotranspiration, and moisture recycling. In aggregate, these limitations may hinder the ability of coupled models to realistically capture the land-atmospheric interactions and thereby limit their skills to predict hydrometeorological extremes at the S2S range. The proposed initiative will benefit NOAA and water enterprise over the western US in the following respects. First, the introduction of new snow parameterization schemes has the potential to improve S2S forecasts of snowpack, and springtime runoff for states heavily reliant on snowmelt runoff. Second, the hindcast experiments, to be conducted for two recent episodes for which operational models performed poorly, will help determine the predictability of these events that can be gleaned through the improvements. |
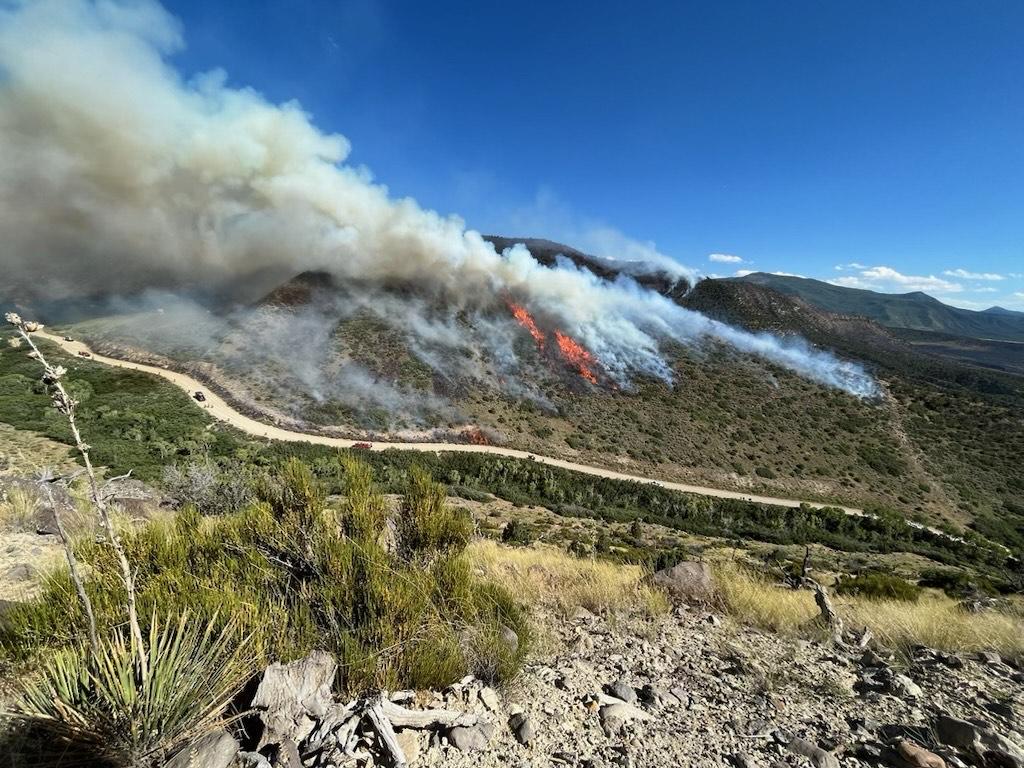
Program Area III: Fire Weather Subseasonal-to-Seasonal (FW-S2S) Forecasting
The increase in the frequency of drought and hot-dry-windy conditions over the last several decades, combined with the continued expansion into the wildfire-urban interface region, has led to a marked increase in the number of acres burned by hazardous wildfires. The number of wildfires and the acres burned are projected to further increase as the climate warms. Wildfires threaten several ecosystems, damage buildings and communities, with downstream impacts on human health associated with smoke and the resulting poor air quality, costing the Nation billions of dollars a year. Projects in this program area support the development of a unified modeling approach for prediction of extreme fire weather and its associated drivers at extended time ranges, focusing on improving fire-weather forecasting capabilities at the subseasonal-to-seasonal time scales in the UFS.
The two projects in this area will receive two-year awards via cooperative agreements with $1,600,906 total funding from the AQFW Program.
*Award totals are distributed over the life of the projects and conditional on appropriations
Projects Selected
Project Title | PI’s /Co-PI’s | Project Description |
---|---|---|
Improving Subseasonal to Seasonal (S2S) Fire Emissions and Weather Forecasts | Daniel Tong (GMU) PI Li (Kate) Zhang (CU Boulder) PI | This project will develop a new ensemble emission dataset to improve the predictability of wildfire emission and fire weather at S2S time scales. Detailed deliverables include: 1) wildfire emission ensemble; 2) S2S wildfire emission prediction model; 3) improved S2S fire weather modeling capability; and 4) peer-reviewed papers. The objectives of this proposed study are to improve S2S wildfire emission and subsequent fire weather forecasting. We will build a new dataset of emission and FRP ensemble that combines the emission information from both top-bottom and bottom-top emission products. Also, we will build a new biomass emission diurnal cycle climatology based on geostationary satellite observation and previous studies.Intended beneficiary: a) NOAA Global Systems Laboratory who is leading NOAA’s development on the S2SForecasting with the UFS. b) NOAA NWS National Centers for Environmental Prediction (NCEP) who will provide guidance for research to operation (R2O) transition.c) This work also contributes to the World Meteorological Organization Vegetation Fire Smoke Pollution Warning Advisory and Assessment System (VFSP-WAS) Initiative. |
Subseasonal Predictability of Fire Weather Metrics for Decision Support | Samantha Kramer (Sonoma) PI Ben Kirtman (Univ. of Miami) PI | We propose determining the degree of success in predicting subseasonal fire weather metrics (HDWI, wind, humidity, soil moisture [as a proxy for fuel moisture], precipitation, and temperature) by using the existing retrospective forecasts from UFS S2S and SubX models. We will also evaluate the impact of calibration, statistical downscaling, dynamical downscaling, and multiple ensemble members on forecast skill (accuracy, reliability, and uncertainty) and usefulness for decision-making with stakeholders. The proposed work will compare two NOAA-sponsored subseasonal forecasts and assess the cost-benefit of different post-processing procedures. Forecast data will not only be evaluated for skill, but will also be used in a case study comparison with sub-daily wildfire growth. Example forecast products will be presented to stakeholders to guide future development of this work for use in real-time decision support and direct stakeholder participation. The evaluation, calibration, and downscaling protocols will be specific to fire weather applications, but may inform others, such as drought indices and extreme heat. The proposed work directly meets the competition priorities to investigate and address uncertainty, assess and improve data processing, and representation of uncertainty in fire weather forecasts to meet operational needs to predict fire weather conditions in the United States. |